Top 8 Cyprus Fintech Companies Revolutionizing the Market: A Deeper Dive
What companies will shake the Fintech scene? Find it out in our newest ranking and learn what is winning the industry right now.
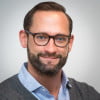
An insightful conversation with Sebastian Niehaus, CTO at SEKASA Technologies, about the transformative role of AI and machine learning in the fintech sector.
In my recent conversation about the fusion of artificial intelligence (AI) and machine learning in the finance sector, I had the privilege of steering the dialogue with pointed questions. Guiding our deep dive was Sebastian Niehaus, a Machine Learning Engineer specializing in Quantitative Finance and the CTO at SEKASA Technologies . Sebastian’s vast expertise provided enlightening insights into the growing symbiosis of AI and fintech .
During our CTO chat, Sebastian and I delved into the world of AI and finance. Despite being miles apart, the conversation flowed effortlessly!
market advantages.
Finance is all about analysing and processing data. Regardless of whether we are talking about payment providers, investment firms, banks or market makers. Every market participant, no matter how small, carries out analyses with existing data, perhaps not with fancy algorithms but with other forms of analysis.
The problem with data, however, is that independent data points are usually useless, they only become interesting when context is added. This context can be added by comparing the data of the current case to similar cases or adding more related and unrelated data. This could be for example the integration of other markets or environmental data in investment decisions or a wider range of transactions in fraud detection.
Jakub: Looking from this point of view – what are AI and machine learning in general?
Sebastian: Artificial intelligence (AI) is the development of computing systems capable of performing tasks that normally require human intelligence, such as learning, reasoning, problem-solving, and decision-making.
Machine Learning is a subset of AI that focuses on training algorithms to learn patterns and make predictions from retrospective data to fulfill a specific task. Thus, it uncovers patterns and mechanisms to automate tasks or generates new knowledge about them.
AI or machine learning methods became that popular over the last few years because they are able to process a large amount of different data features. That is a large difference from the classical statistical models that we are using in finance since the 80s.
Jakub: That’s an interesting insight! So, what are the benefits of machine learning for FinTech companies?
Sebastian: In one sentence: They are using their full potential!
FinTechs are pure data producers and they have to deal with large amounts of financial and alternative data. Out of this data, they can discover new business potentials, secure current processes, make their decisions more transparent and improve the quality of their decisions.
Even if processes or especially decision processes are clearly defined and well working, it often makes sense to add machine learning algorithms to provide a second view and reduce the subjective error by humans. This could, for example, prevent investment companies from making FOMO investments.
Jakub: What is the motivation and business case for integrating AI?
Sebastian: It is often about securing competitive advantages, process optimization, or just answering specific questions. In addition, there are also topics such as future viability – which is an issue for very established financial companies, for example. These companies often don’t even know what potential lies in their data and come with the simple request: “We’d like to try out what can be improved with Machine Learning in our company”
Let me make the answer more tangible with a few examples:
I can continue this list forever:
Jakub: Let’s expand more on that topic – what are some popular use cases?
Sebastian: Some of the most used ones are:
Jakub: How can machine learning be integrated into FinTechs?
Sebastian: It is important to start with the business case and the current processes. This seems to be obvious, but it is common that this point is completely underrated and often missed.
Jakub: Looking at your experience – how difficult is it to integrate machine learning into FinTech companies’ processes?
Sebastian:
This may sound like a lot, but many of these tasks can be standardised and automated. And once integrated, the benefits quickly outweigh the effort.
Jakub: What you have mentioned earlier begs for a question – how adaptable are machine learning models to changing environments?
Sebastian: Machine learning models are highly adaptive and must be able to respond quickly to changing environments. They can be adapted to changing environments through techniques such as retraining on updated data, transfer learning, and continuous monitoring. This ensures that the performance of machine learning models remains relevant and accurate over time.
Example: For Trading companies.
Trading signals for forex or commodities can change for example through changes in the behavior of other market participants. We can see this for example through the rise of trading apps, but also through more advanced events.
For this reason, companies that are already working with quantitative methods should always consider keeping their algorithms updated and include fresh ideas.
Jakub: Sebastian Thank You for an insightful conversation. We’re excited to see more growth and innovation in this field!
Sebastian: My pleasure, Jakub. I appreciate this platform as it allowed us to discuss the exciting progress in our field and its significance in the ever-changing digital landscape.